For the last few months, I’ve tried to get down to writing about the joys of cold showers. But the words refused to line up. At the same time came a gnawing feeling that I should write about copyright issues in Artificial Intelligence (AI) image generation.
You may think a cold shower is preferable to reading about copyright and AI. And you may be right.
Last year I wrote on AI and photography: if you’d like an introduction to the subject, that’s a good place to start.
But copyright wins.
Here’s why. I see a great deal of anger, outrage and anxiety in artists over the way AI (actually Large Language Models – LLMs) like DALL-E or MidJourney or Stable Diffusion have used copyright work. We know that millions upon millions of copyright works have been ‘scraped’ to develop these models. (Doesn’t ‘scrape’ have the derisive connotations many think the process deserves?)
We know that no prior permission was obtained for their use. And we know these companies are earning billions – if only in capital raised – from their models. If you’re an artist, photographer or designer, you may be so outraged, you want call the nearest lawyer to sue the hell and high water out of these exploitative corporations. And several have done just that.
It’s obvious the AI companies are in the wrong. So what’s there to lose?
My answer is: everything. Even if you have the exceptionally deep pockets to hire the calibre of intellectual property lawyer needed, my view is that you are unlikely to win. Instead of sharing the delights of cold showers with you, then, I will share my reasoning for what is, without any doubt, a view that is deeply unpopular amongst creators. If you’d like a thread to guide you through this jungle, it’s this: ontology outruns legality.
Let’s look at today’s popular views on the subject.
1 AI infringes styles of art
Photographers and illustrators across the globe are flabbergasted to find their particular styles of creating work are being copied for free by thousands of keyboard trampers. Or they’re furious that their special style – one spent years perfecting – could be imitated by a free web app. If, however, they had learnt any copyright law in college, they seemed to have clean forgotten it all. Copyright law does not protect art styles. Never has, never will.
On one hand, the evidence is ample: AI generative models expressly invite users to specify styles from contemporary artists, as well as classical ones such as Picasso and Monet.
On the other hand, the simple fact is that copyright protects works of art that are fixed in a tangible medium: a painting, photograph or illustration on paper, and the like. Even a digital file on computer memory is protected. That’s because ‘tangible’ in copyright not only means you can touch or pick it up, but that it exists in some stable form that you can work with.
It follows the style of an art work receives no protection.
One reason, the logical and legalistic one, follows directly from the tangibility requirement: a style cannot be fixed in a form independent of an individual work so it cannot be protected. That is, indeed, the point of distinguishing the style of a work or performance from the work itself. You can describe the style, get as ecstatically ekphrastic as you like, but it’s still not something you can make tangible as an artwork. The words are a meta-art. You can only make a work of art that mimics the style.
Descriptions like ‘eyes on same side of face, big noses in profile, simplified outlines and flat colour’ may describe one of Picasso’s styles. But can that be protected? One of my photographic projects has a very distinctive signature: they are very pale with washed out colours, and simple shapes. But can I copyright this style? Of course not. Why should – or how could – anyone be stopped from making over-exposed images of simple shapes?
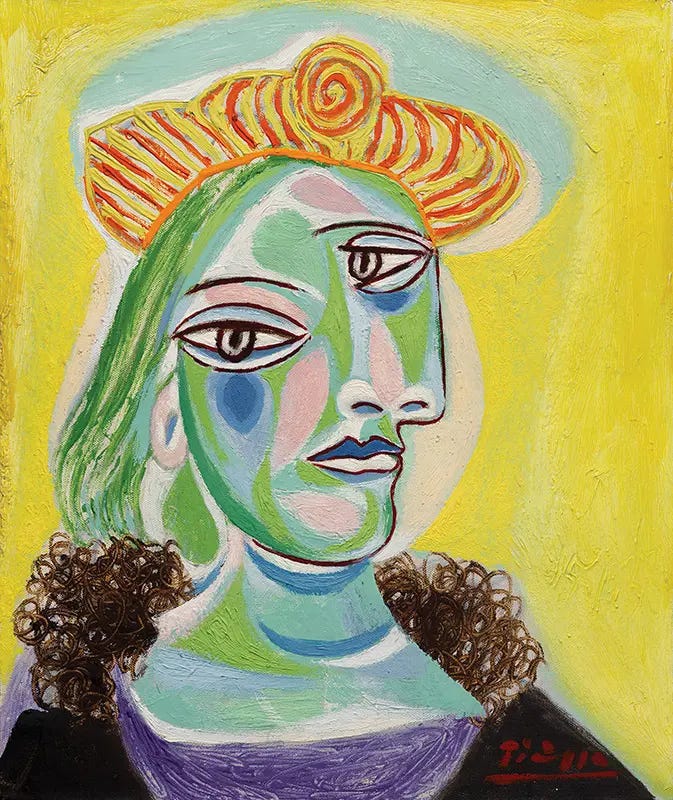
That brings us to the second reason why style has no copyright protection. It’s good policy.
Style has no protection because it should not. Generations of artists have learned their craft by copying masterworks. I used to teach photography at university. One of the standard exercises for students was to find a classic image they admired and analyse how it was made, then to create a photograph matching all key qualities while not copying the subject of their seed photograph. Part of the test was to see whether viewers could recognise the photographer their work was based on. Were the students infringing on copyright? No; there is nothing for them to infringe. Did one of them infringe copyright when she thought ‘Hey; I like that. I’ll develop that!’ She did what thousands of students in visual arts of all kinds have done for centuries. Did they all infringe copyright? (There’s an issue of scale; I’ll look at that later.)
2 Copying a copyright work is (not necessarily) illegal
One of the howls of anger aimed at LLMs grew quickly into a planet-sized cyclone. Almost overnight, artists worldwide woke up to the fact that millions upon millions of their works (at least 200 million images by some counts, perhaps up to 400 million) had been served up to feed AI’s insatiable appetite for data. That is copyright infringement on a gigantic scale. There was a cry of rage of unusual unanimity.
Let’s examine this.
In the care-free days of physical artworks, you unequivocally could not copy a work. It was simple: a copy of a painting was a painting, a copy of a book was a book. That changed in the 1950s when images and music were began to be digitised. It meant that a copy of the artwork was created and held for a time in some apparatus, whether a computer, drum scanner or magnetic tape. Thus, as the laws of various states stood at the time, all these digital copies were infringing if it wasn’t the copyright owner doing it. Ontology – the existence of a new thing – outran the law that can legislate only for things known to the law.
I remember when flat-bed scanners became widely used – in the late 1980s – users were blissfully unaware that their gleeful scanning old photos, books, paintings that didn’t belong to them was illegal.
As you probably know, out of the resulting mess, two doctrines evolved. One was to provide for certain exceptions: your copying of works is okay provided, for example, it was for private study. This is the doctrine also called fair dealing, or fair use.
The other strand was the recognition that, for certain technical processes, it was necessary to hold a temporary copy. If you’re a printer and a client sends you a third-party file for printing, it can’t make sense that you infringe copyright of the image by holding a copy in your computer. It’s what EU law calls ‘temporary acts of reproduction’.
One of the many criticisms levelled at the training of LLM such as OpenAI, Stable Diffusion and others is that they must have copied millions of works without permission. They did. No question. But was the copying illegal? Not if they were temporary acts of reproduction for the technical purpose of training a LLM. (More on this below.)
3 AI generators use a database of copyright works
We reach one of the most widespread and deeply-held beliefs. It is that LLM hold a vast database containing the zillions of images stolen from the Internet. To put it bluntly, that’s wrong. Anyone with computing background knows that it would be impractical, impossibly clumsy and plain bad computing practice to store millions of images in order to generate new ones.
Given that everyone now knows about how images are composited in image manipulation apps, it’s natural to think that AI generation works in a similar way. So you start with a prompt ‘Polar Bear crossing Times Square in Art Deco style’. The process of generating images involves searching all, say, 200 million, images to find the dozen that correspond to ‘polar bear’ and the scores that show ‘Times Square’ and dig up some images of Art Deco. For the AI to generate image it must extract bits from existing images and somehow plaster them together to make an generated image or synthogram. Therefore, goes the reasoning, LLM must access to a big database of images.
Even without a computing background, you may be able to guess (feel) that searching through zillions of images to paste together the various components would be an awfully inefficient, long-drawn out process. But it is how we humans do it so it’s worth giving this a little attention.
For the polar bear, you would search through pictures from your trip to Antarctica (lucky you!). You find a shot or two showing the bear plodding over the snow. You put them aside while rejecting all the others – swimming, standing on two legs etc. What you are doing is looking for a particular bear picture that corresponds to an idea you have in our head of what the final image will look like. That idea in your head is roughly what AI engineers call the ‘latent space’.
Then you’d check your pictures of a trip to New York. Against the ideas you have for the final image, you reject all the night shots but choose daylight shots. However, there are no shots looking along the road. So you to trawl through dozens of other pictures to find a shot of a road you can use. Next you have to composite these elements in the style of Art Deco. For that you may need some art references too. Sounds like a full day’s work!
Now here’s the thing. Image generation actually does go through similar searches to arrive at its output. But what LLMs don’t do is to work with pictures. As we’ve said, that’s far, far, too clumsy and slow; the engineers describe such data as too high dimensional. Computers work with numbers, and they like the crunchy stuff organised into matrices. To say again: when generating images, LLMs do not work with images.
They work with tensors. Here is the outline for a nine-dimensional tensor. Yes; tensors are multi-dimensional, and they use not only scalars (like RGB numbers) but vectors too:
Here we poked our fingers into what the industry calls the black box. Physics and maths majors know and love tensors. But I’ve mentioned that tensors are multi-dimensional matrices with vectors, and even second time around, most readers won’t be any the wiser. Welcome to the black box. What matters is that computers will munch merrily on tensors but will whine all day if given a slew of JPEG files to work on.
Roughly speaking, tensors are to JPEG files what JPEG files are to prints on paper. A JPEG file of a print is only a partial representation of the original but it is easily sent around in a computer, making it easy to manipulate, use, store, share. Totally unlike the print that exists essentially in only one form. So are tensors partial representations of JPEG files.
Tensors of a JPEG file also represent only some of the properties or high-level features of the image, such as position and orientation of edges, general shapes, colours and gradients. Tensors are a representation of the visual information in an image in matrices. Think of a Lego set for a castle: it contains all the bits needed to make the castle. But the same bricks can be combined in other ways to make a different castle or even be turned into a car with the addition of blocks from another kit. The individual bricks are like tensors extracted from images. And as you can guess, many tensors could be – like Lego bricks – almost identical with each other such as those for pupils of human eyes.
Tensors are the raw ore that the LLMs mine from tons of images: this is deep learning. Almost literally, images are ground to dust and any precious flakes are extracted for refining as tensors. That includes not only visual content but relevant text data such as the title of the image, where and when it was made (but probably not copyright information). The resulting pieces – the tensors – can mathematically processed to be compressed, combined and recombined just like Lego bricks.
In short, the training of LLM on images stores information that bear no resemblance to images. It is a change of modality; LLM training delivers a computer-readable ekphrasis – a description of images – with no literary but great computational merit.
Side note: fair learning
The way LLMs mine specific bits of information from a big mass of images may remind our older readers of their student days. Those of us who grew up and studied during the early days of photocopying will surely recall the joys of copying huge numbers of pages from books and journals for study. We would make copies of far more than we could possibly read. But it was never the intention to read them all. The intention was to scan the articles for juicy bits, for insights that helped write the essay, to provide short-hand notes for key facts and references. In other words, we copied an entire article not in order to consult or read all of it, but in order to extract the tiny fraction needed for an essay or a test. And it saved the bother of checking out a vast tome from the library when all we needed was one chapter.
And even now – be honest now – let’s review all the PDFs and ebooks that you download. How much do you actually use? Most often (I’ll bet) from all that downloaded stuff, you clip a sentence from the summary and a bit of the conclusions; 99% of the rest you don’t read … and don’t need. The point is not that we’re making the copy for study purposes so it’s okay (a fair dealing exception), but in order to examine the reason for the copying.
But the whole had to be accessible for the desired portion to be extracted; that’s the heart of the issue.
We want to extract key elements, while ignoring everything else. That is precisely the training of LLMs. The difference between a hardware farm of thousands of computers and you is principally one of scale. Uploaded images are preprocessed by scaling down usually to as small as 224x224 pixels then their features are extracted according to the training schedule.
Suppose it’s ‘eyes’ today. We feed the learning circuits with pictures of cats, dogs, pumas, alligators, humans. The LLM learns the general features of eyes. After looking at many different eyes, the system learns to recognize the similarities between all eyes, simultaneously, it’s learning the differences between cats and human eyes. The key point is that the system is not copying any individual pictures of eyes in its ‘study’ of eyes: it’s extracting key elements, while ignoring everything else.
This is not the place to explore the reasons why a statutory recognition for fair learning or fair training – copying for the purpose of extracting elements or components – could help regulate the use of datasets for LLM training. Again, the emergence of new practices leaves the law panting to catch up – ontological innovation render statutes out-dated. But let’s turn from that pretty little jurisprudential side-path and return to other views on AI image synthesis.
4 There is no creativity in generating images with AI
One of the most trenchantly held views – held tight with the force of a true co-dependency – is that there is no creativity in the generation of images by AI. There’s no exercise of human genius. This view has received institutional support from the US Copyright office in its rejection of copyright registration in the AI-generated images in the ‘Zarya of the Dawn’ case. (See this report, for example.)
To put my cards on the table, I think the US Copyright office is right; and it’s also wrong.
Within the context of current law and understanding, it’s clear enough that throwing a few words into the black box that runs up an image within seconds involves no skill, no art, and no creativity from the user; the resulting image is merely a product of a machine. Well, guess what? That’s been the legal perception of new art forms throughout history.
Gutenberg printed the first mass-production books in the mid 1400s. It wasn’t until two and a half centuries later that such books received protection in law, in the Statute of Anne 1709.
Dürer invented engraving in the late 1400s. The process evolved into the preferred method for reproducing line art works but remained unprotected by law for centuries. Not until 1735, after heavy campaigning from the likes of Hogarth, did English law recognise engraving as an art worthy of legal protection.
Photography was invented in one form (a dead end) in about 1816, then as Daguerreotypes in 1839, and as Calotypes in 1841. It wasn’t until The Copyright Act 1865 that US courts recognised photography as producing copyrightable works. Even so, doubts surfaced in 1883 about the legality of the Act. This went all way to the Supreme Court that found photography was a kind of writing, therefore falling into the constitutional framework. And despite legal protection, photography fought for a cultural battle for a hundred years to be recognised as an art that required human creativity notwithstanding its dependence on a black box that did much of the work.
You see the pattern.
Most users have no idea what is going on when they hit ‘Enter’ after typing a few words an AI prompt box. A few seconds later, a picture pops up on their screen. How on earth did that happen? Millions of users don’t know, nor do they care. After trial and error, they learn how to obtain the results they want. Well, that was easily said: users report drafting hundreds of prompts before they see results they want. Some claim a lot of creativity goes into learning how to get the machine to work properly.
The AI is in fact training its users how to transfer a visual idea they in their minds across the communication gap mediated by screen/keyboard into the AI model.
The parallels with photography are obvious: the camera is literally a black box that works in ways beyond the knowledge or attention of most users. And all users learn quickly that only certain – almost canonical – ways of using a camera produce results they like.
And now that cameras have super-hero abilities, users don’t need the fluffiest clue how to use them. It started with the Box Brownie and continues through to the cameraphone. Photographers scorned Brownie users as not ‘real photographers’. Each technological advance – from auto-exposure to colour to auto-focusing – has been scoffed at for not needing skill therefore degrading of the art. Even now, photography snobs are contemptuous of cameraphone photos. There’s some truth in that. It’s so ridiculously easy to make an image, ability is now predicated on a meta-photographic skill: curation.
Successful photographers are skilled a choosing images. Success with AI image synthesis calls for a parallel skillset.
Within months of their introduction, users of DALL-E, MidJourney and StableDiffusion clocked up millions of images generated, with over 15 billion images generated as of early 2024 – a mere two years after DALL-E 2’s debut. AI users discard hundreds of synthograms or AI images before they obtain an image they want to use. Hello! And how many images do photographers discard before they’re identify a ‘keeper’?
What is ‘prompt engineering’?
A quick reminder of the user experience of AI image generation. You log in to an appropriate account, enter a text describing the image you want to see, click ‘send’ or ‘enter’.
You may be very general with your prompt e.g. ‘Apple held by child’ or quite specific ‘Golden Delicious held in left hand of curly dark-haired 3-year old girl wearing a green shirt in animé style 1:1 format’.
Then you wait. After a while (depending on how much you’ve paid for the service, time of day, etc.) one or more images appear on your screen. Most likely, you’ll want something changed in the image, so you edit your instructions for another iteration.
The result is that users of AI have had to develop a brand-new, never before-seen skill. It quickly gained the name ‘prompt engineering’. It’s the technique of composing a prompt that efficiently ensures the AI generates the images you want; it’s about leaning how to ‘communicate’ with and ‘guide’ the AI.
For many users, prompt engineering involves the activity we associate with creative skills. It needs appropriate knowledge, practice and application to develop. Skilled operators who understand how to parse prompts obtain consistently better results than unskilled. New ways of phrasing prompts can synthesise new kinds of synthograms.
Nonetheless, it remains true that it’s the LLM that does the heavy lifting. While the role of users is to tell the LLM what to lift, we can’t tell it exactly how or control exactly what is lifted. How much does this differ from using a camera? It’s not as much as you might think. While photographers control a few functions of the camera, the camera – the black box – gets on with a great deal of other business in the background.
The burning question is: to what extent must a user of a tool be in control for the resulting work to qualify as a human creation? This matters as in many legislations as the answer determines whether anything in the work can be protected. Nothing definitive yet, and I doubt there will be.
5 Is there copyright in AI generated work?
The question of whether copyright subsists in synthograms or AI-generated images wobbles atop two inter-twined elements: agency and, naturally, that of creativity.
In our context, ‘agency’ means the person, machine or system that does the work, like generating the picture; the ‘author’ in copyright parlance. Where you stand on this issue neatly divides believers from haters. Believers consider that they are the agents making an image; the AI is only a machine, even if it is one that maddeningly doesn’t do exactly what it’s told. So the human agents expend a great deal of skill and effort in prompting the machine work properly.
In the other corner, the haters of AI consider that the agent is surely the AI model itself – a vast computer thingy running in enormous processor farms. One of their argument aims precisely at the problems prompters have in getting the machine to produce the desired image. A few hours of AI image generation will show you that the machine appears to have a mind of its own (a phrase chosen with some care). You can keep asking for two hard-boiled eggs and the LLM may offer a hen, a poached egg, five eggs, a stove top … anything but two eggs.
Therefore this fulfils a criterion of agency: LLMs appear to ‘think’ for itself. The fact that the precise same prompt given twice always produces a different image is more evidence of AI’s agency. (Indeed, you have specifically to train a model to produce identical images from identical prompts.)
The state of play is that the question is not settled.
One line – following UK copyright law – is that agency is interpreted as the person ‘by whom the arrangements necessary for the creation of the work’ are made. Note that this provision is specifically for ‘work which is computer-generated’ (Section 9 (3): very prescient of the drafters!). This aligns with the doctrine that the person who commissions a work, or who directs a crew, may be regarded as the author of the work. The director is the agent, the people she’s directing are the ‘machines’ doing the work for her. This applies, for example, in film production where the production company or director may allow the camera operator some freedom to frame, pull focus, or zoom yet no-one expects the camera operator to own the film’s copyright.
This raises the issue of how much and what quality of creativity would qualify a work as creative. It’s obvious there’s a viper’s nest of issues here: from whether a machine can be truly creative to the fact that some legislations do not require a creative human input to grant copyright protection anyway. Let’s move on, hurriedly, to some kind of conclusion.
6 So, is AI infringing our copyrights?
My view is that copyrights in works used in training LLMs for deep learning have not been infringed because current laws do not forbid what was involved. It’s a clear case of ontology outrunning legality. The way LLMs consume copyright work is new sui generis and is simply not covered by existing statutes.
This doesn’t mean the gobbling up of billions of bits of data is right in an ethical sense. It’s perfectly clear – certainly with the blinding hindsight given by the howling push-back – that companies such as OpenAI could or should have put some provision aside to compensate for using copyright materials for training, even if (as I am sure) their lawyers told them ’Nah; it’ll be alright. What you’re doing is so new, ain’t no law stoppin’ ya.’
The players arriving later in the game, such as Adobe, Shutterstock, Getty have learnt from the public relations disaster when everyone realised how many zillion items were used to train LLMs. These companies offer models of returning revenue to their contributors when their work is trained for AI use.
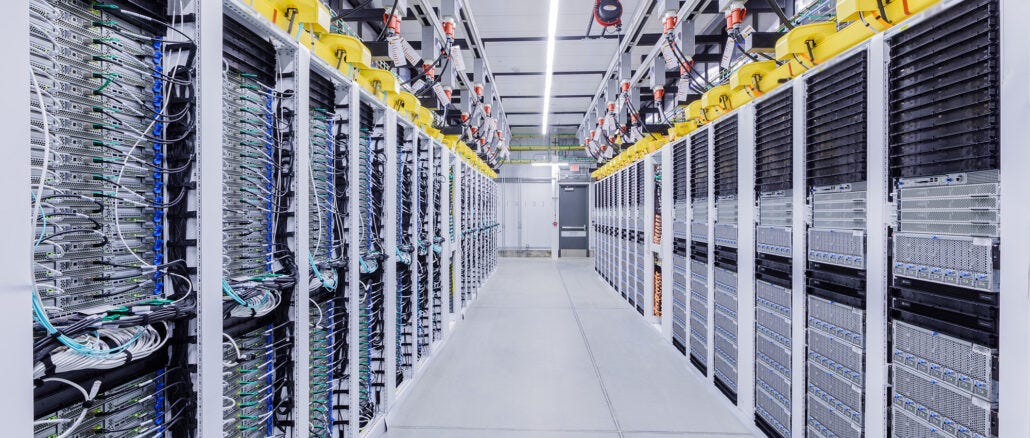
Mind, it’s only fair to examine the costs of creating, training and running these models. Some estimates put it at $5 million to train, using a million or so training hours costing over $3 million plus capital computing costs. To service say 100 people at any one time needs around 8 GPU (Graphic Processor Units) – around $10 million of computing plus 6-figure daily running costs. Current estimates put the number of active users of ChatGPT at around 100 million who made 1.6 billion visits in December 2023 alone serviced by some 30,000 GPUs. As for daily running costs including salaries for top (human) brains to run the machines daily burning up more than $100,000 in electricity, a figure of $700,000 is in circulation.
Summing up … for now
We all know about the truly explosive growth in the adoption of AI in all fields. From medical imaging to share dealing to assassination planning, AI is part of our lives whether we like it or not. It’s only three years since the first wavelets of the AI tsunami first rippled across our screens. Crude, cartoony and clumsy those who laughed at the early attempts were like those who guffawed at the images from early digital cameras. The big difference is that the process of synthesising images is even more opaque than the processes producing the digital image. The mathematics for digital cameras is a lounge lizard to the sprinter of the maths for AI image generation. One result is that hardly any critic of AI actually knows what they’re talking about. And only the unwise would today claim to have any definitive word on this technology.
I’ll say it again, however: ontology has outrun legality. New technologies scampering wild have left the statute books covered in dust. If only AI companies had shown more respect for the building blocks of their technologies, if only they had engaged in more education, and if only key actors had shown more interest in using the tech for good instead of their unseemly rush to claim their seam of gold, we would all have benefitted. And the money-grubbers could still make their millions.
My personal position is that AI image generation is an invaluable tool for art; and it’s tremendous fun to use. AI produces synthograms or images by the synthesis of image features held in the LLM; it does not – repeat – does not produce photographs. I do not use AI to produce anything that looks like a photograph. Like any other tool, we can abuse it or we can do good with it. For me, that means using AI tools to make art that delights, entertains, inspires, challenges or questions … all the things art is for.
So many well-said points here. Thank you for sharing your thoughts about this!
A great article, highlighting many of the issues which much discussion of AI seems to miss.
Just one point though. As someone who has been a director, a cinematographer and a camera operator, your sentence "yet no-one expects the camera operator to own the film’s copyright" struck me as a poor analogy. This is because you wouldn't expect the director to own the copyright either. Normally the copyright would be held by the production company.